Experiments
Visual examples of synthesized images
\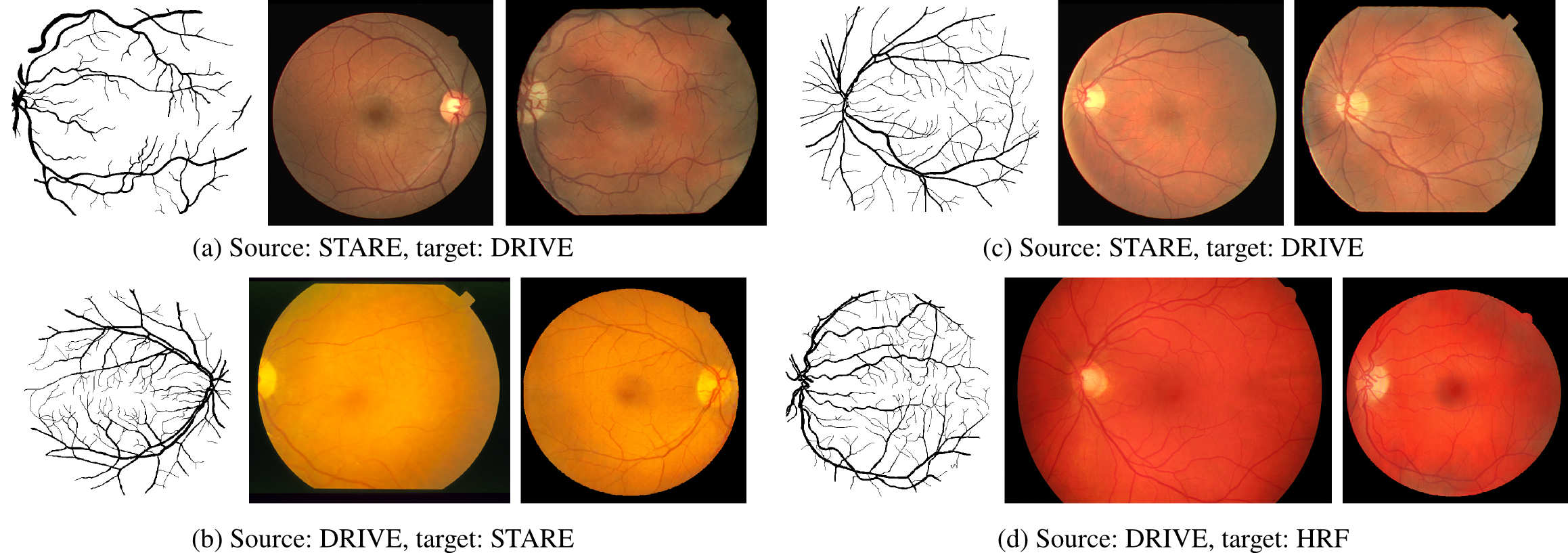
Figure 1: A gallery of synthesized images with different target styles. Each subplot displays a triplet of source vessel structure, the target style and one of the synthesized images: on the left is an exemplar source vessel structure, in the middle is an exemplar target style image; On the right is an exemplar generated phantom having the same style of the target dataset while maintaining the vessel structure of the source dataset.
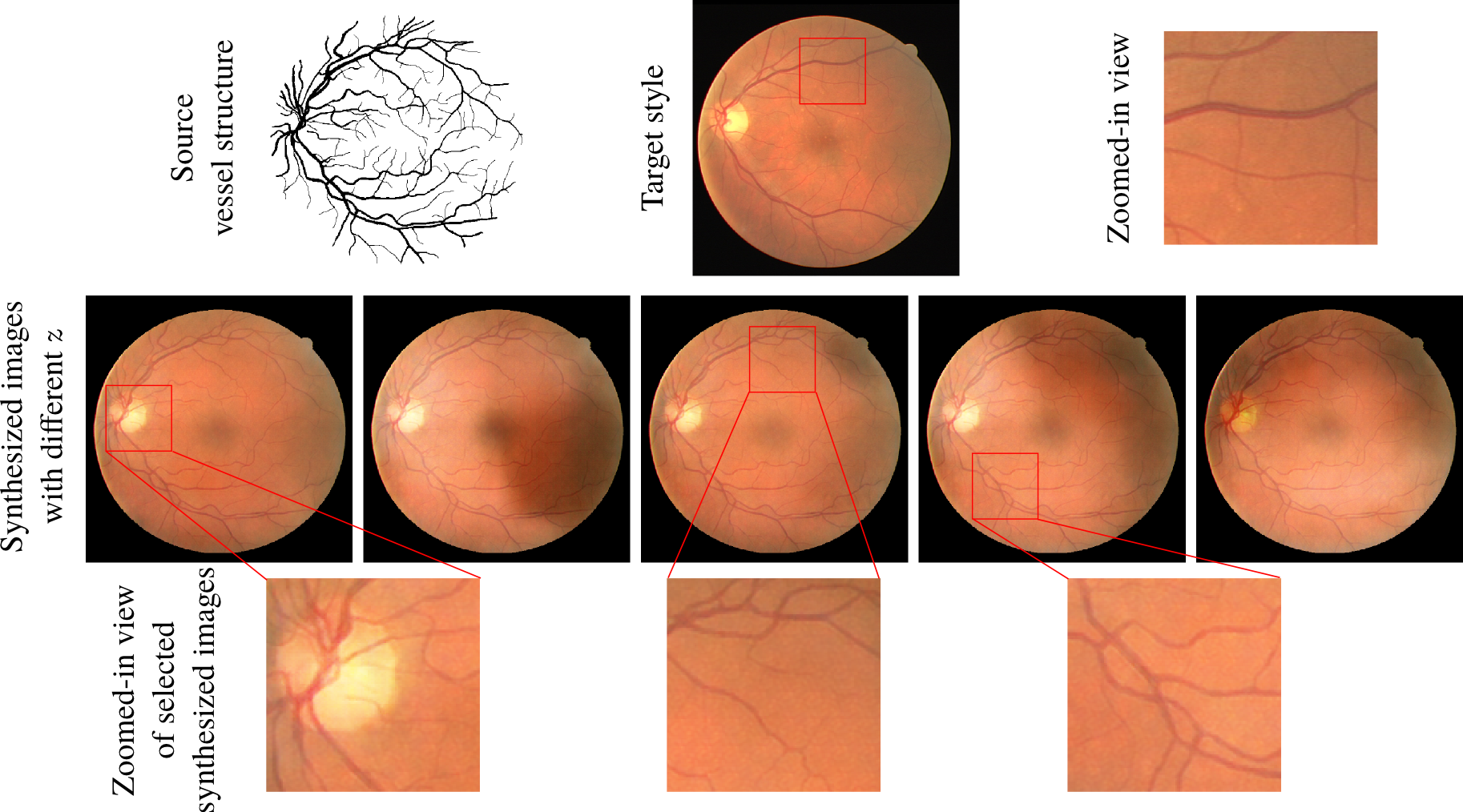
Figure 2: An illustration that distinct phantom outputs are generated from the same source vessel structure but with different noise code z. Top row displays the source vessel and target style. Second row shows the diverse synthesized images. The last row presents the zoomed-in view of selected parts.
Quantitative and qualitative result on benckmark datsets
Table 1: Performance comparison of state-of-the-art methods over a diverse range of target benchmarks. Segmentation methods include the unsupervised MSLD method, the Pixel-CNN and the DRIU models trained on DRIVE or STARE datasets respectively, as well as our approach. Results are reported in F1-score (\%).


Figure 3: Precision recall curves of the DRIVE, STARE, HRF, and IOSTAR datasets as the target. By default, the DRIVE dataset is the source dataset, with the exception that when DRIVE is the target dataset, STARE is used instead as the source. DRIU is the supervised segmentation method engaged here in our approach.
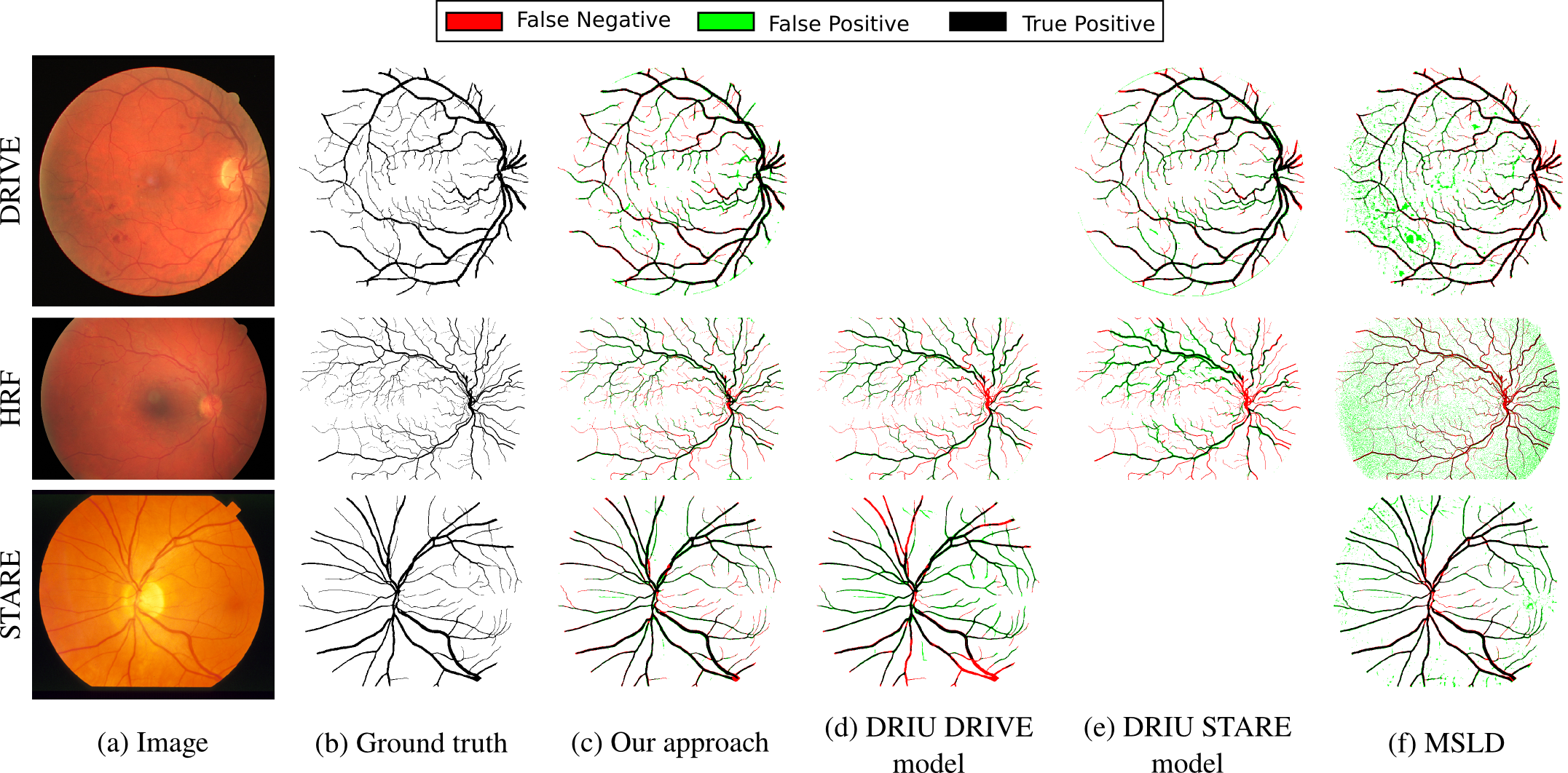
Figure 4: A visual comparison of segmentation results over different datasets. (a) Input fundus images from various datasets: DRIVE, HRF and STARE; (b) Ground truth vessel structures; (c-f) are the error maps of the comparison methods. Note (c) is our approach with DRIVE being used as source dataset, except for the first row where STARE is used as source instead. (d-f) are the supervised/unsupervised comparison methods trained on DRIVE and STARE datasets, respectively. DRIU is the supervised segmentation method engaged here in our approach.
Qualitative results on un-annotated datasets
Anzhen and Kaggle datasets
Smart phone based image dataset