Method
In this work, we aim to solve the problem that segmenting new target retinal fundus images with dissimilar source training set. We present a two-step supervised learning approach . The pipline of our method is shown in Figure. 1. The first step focuses on the construction of a synthesized dataset based on the target query images. The second step proceeds to learn a supervised segmentation method based on the synthesized dataset. The constructed dataset is capable to bridge the gap between the existing source dataset and the new target query dataset. This is the crucial part of our approach. The technique used to build the synthesized data is recurrent generative adversarial network (R-sGAN) whose details are shown in Figure. 2. With the help of R-sGAN, the realistic-looking training images can be generated containing the content of source dataset with the same textural style of the target query images. The model structure of our R-sGAN is displayed in Figure. 1(a) with detailed GRU structure depicted in Figure. 1(b). The involved loss fuctions are summarized in Figure. 1(c). Figure. 1(d) presents the network used for feature extraction.
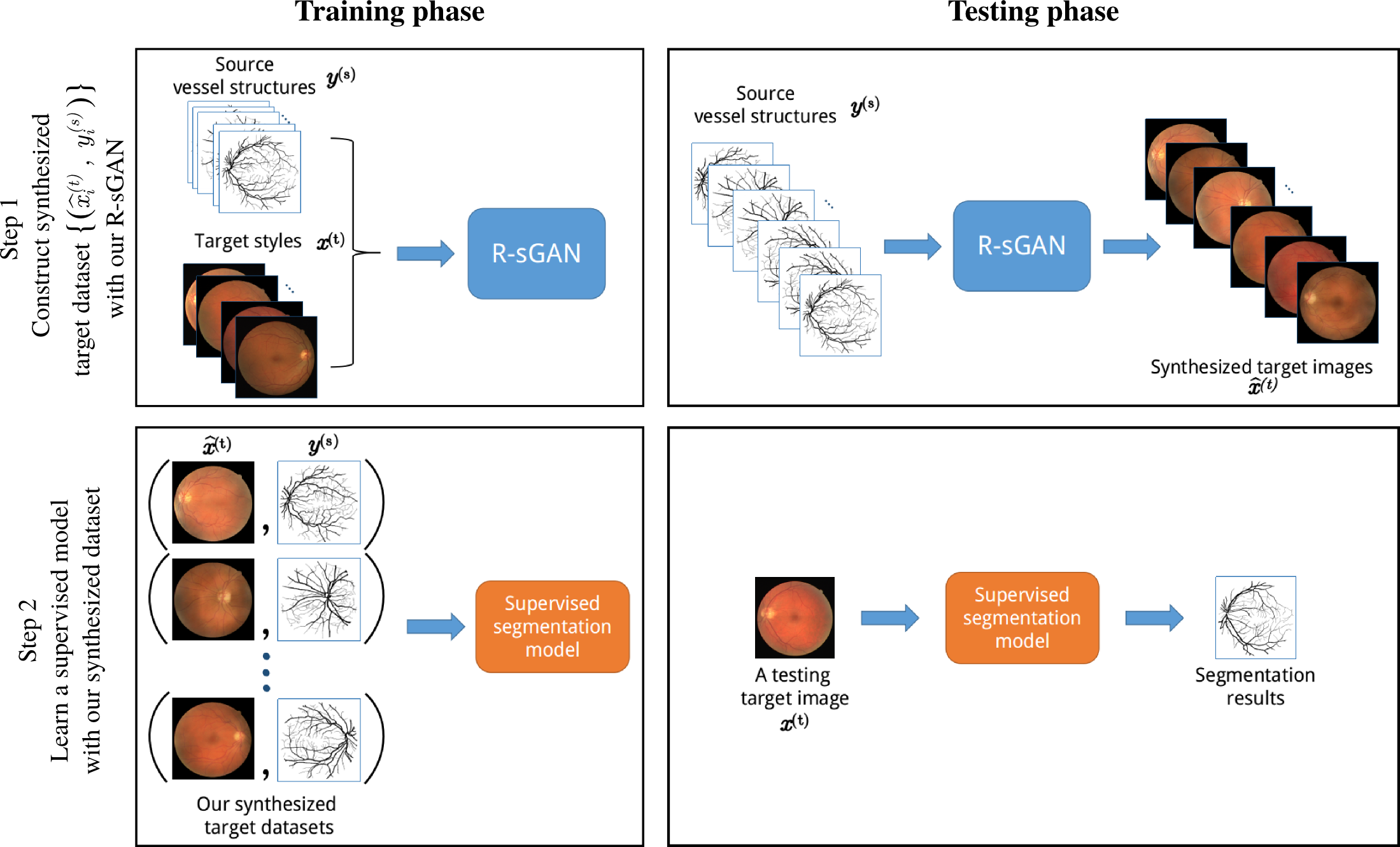
Fig. 1 The flowchart of our approach that contains two steps. Step 1 involves the construction of synthesized target training set that has the vessel structures of the reference training set and the textural appearance of the target query images. Step 2 thus engages a supervised segmentation method to learn a dedicated target model.

Fig. 2 (a) The proposed R-sGAN synthesis process, where the GRU-type component is utilized and depicted in (b). The involved loss functions are summarized in (c). The generator gate adopts the U-net structure, while inspired by the GAN technique, a corresponding discriminator is considered in (c) that also induces the adversarial loss and the diversity loss. The style and content features considered in our approach are directly obtained from the deep learning representation. Without loss of generality, the VGG network is considered in our work to extract these features, which is illustrated in (d).